Joan Cornet, Director Digital Health Observatory and Coalition of the Willing at ECHAlliance, identifies some of the barriers to implementing Artificial Intelligence in Healthcare. This is Part Two in a series of articles. Read Part One.
In Star Wars: The Empire Strikes Back, Luke Skywalker is rescued from the frozen wastes of Hoth after a near-fatal encounter, luckily to be returned to a medical facility filled with advanced robotics and futuristic technology that treat his wounds and quickly bring him back to health. Of course, that’s the stuff of science fiction … for now.
Back in 1950, Turing argued that for a genuine AI we might do better by simulating a child’s mind than an adult’s. This insight has resonance given recent work on “life history” theory in evolutionary biology—the developmental trajectory of a species, particularly the length of its childhood, is highly correlated with adult intelligence and flexibility across a wide range of species (1)
Many researchers and companies have tried to apply artificial intelligence to the healthcare system, with applications including image interpretation, voice recognition, clinical decision support, risk prediction, drug discovery, medical robotics, and workflow improvement. However, several important technical, ethical, and social barriers must be overcome, such as overfitting, lack of interpretability, privacy, security, and safety.
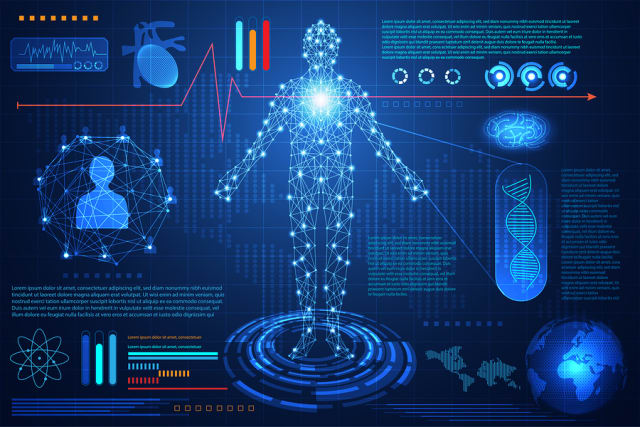
Perhaps because of the enormous complexity AI is still a relatively new technology, especially in the healthcare industry where adoption remains in its infancy. As AI and machine learning tools become more sophisticated, their use cases have expanded; however, adoption of AI remains low. Some of the challenges are:
Doctors make decisions based on learned knowledge, previous experience and intuition, and problem-solving skills. Getting doctors to consider suggestions from an automated system can be difficult. It’s likely that some elements of AI literacy need to be introduced into medical curricula so that AI is not perceived as a threat to doctors, but as an aid and amplifier of medical knowledge. In fact, if AI is introduced in a way that empowers human workers rather than displacing them, it could free up their time to perform more meaningful tasks or grant more resources to employ more workers. Doctors should be prepared to play a key role in applying artificial intelligence through the full course of development, validation, clinical performance, and monitoring (2)
Privacy, while important in every industry, is typically enforced especially vigorously when it comes to medical data. Since patient data in European countries is typically not allowed to leave Europe, many hospitals and research institutions are wary of cloud platforms and prefer to use their own servers.
For startup companies, it’s hard to get access to patient data to develop products or business cases. Usually, this is easier for medical researchers, who can make use of standard application procedures meant to facilitate research based on patient clinical data. (3)
AI algorithms meant to be used in healthcare (in Europe) must apply for CE marking. More specifically, they need to be classified according to the Medical Device Directive. Stand-alone algorithms (algorithms that are not integrated into a physical medical device) are typically classified as Class II medical devices.
The General Data Protection Regulation (GDPR) directives introduced in May 2018 will also lead to a number of new regulations that needs to be complied with and that are, in some cases, not clear-cut. For example, some degree of transparency in automated decision-making will be required, but it‘s hard to tell from the directives what level of transparency will be enough, so we’ll probably need to await the first court cases to learn where the border lies. Other issues are likely to result from the requirement for informed consent. For example, will it still be possible to perform research on dementia under the new regulations, considering some of the participating individuals may not be able to give informed consent?(4)
Despite potential difficulties in establishing parameters, transparency of decision support is, of course, paramount to medical AI. A doctor needs to be able to understand and explain why a certain procedure was recommended by an algorithm. This necessitates the development of more intuitive and transparent prediction-explanation tools. There is often a trade-off between predictive accuracy and model transparency, especially with the latest generation of AI techniques that make use of neural networks, which makes this issue even more pressing. An interesting viewpoint on transparency and algorithmic decision-making is given in a paper named Counterfactual Explanations Without Opening the Black Box: Automated Decisions and the GDPR, which was co-written by a lawyer, a computer scientist and an ethicist. (5)
The latest techniques in AI making use of deep neural networks have reached amazing performance in the last five to seven years. However, the tooling and infrastructure needed to support these techniques are still immature, and few people have the necessary technical competence to deal with the whole range of data and software engineering issues. Especially in medicine, AI solutions will often face problems related to limited data and variable data quality. Predictive models will need to be re-trained when new data comes in, keeping a close eye on changes in data-generation practices and other real-world issues that may cause the data distributions to drift over time. If several data sources are used to train models, additional types of “data dependencies,” which are seldom documented or explicitly handled, are introduced. (6)
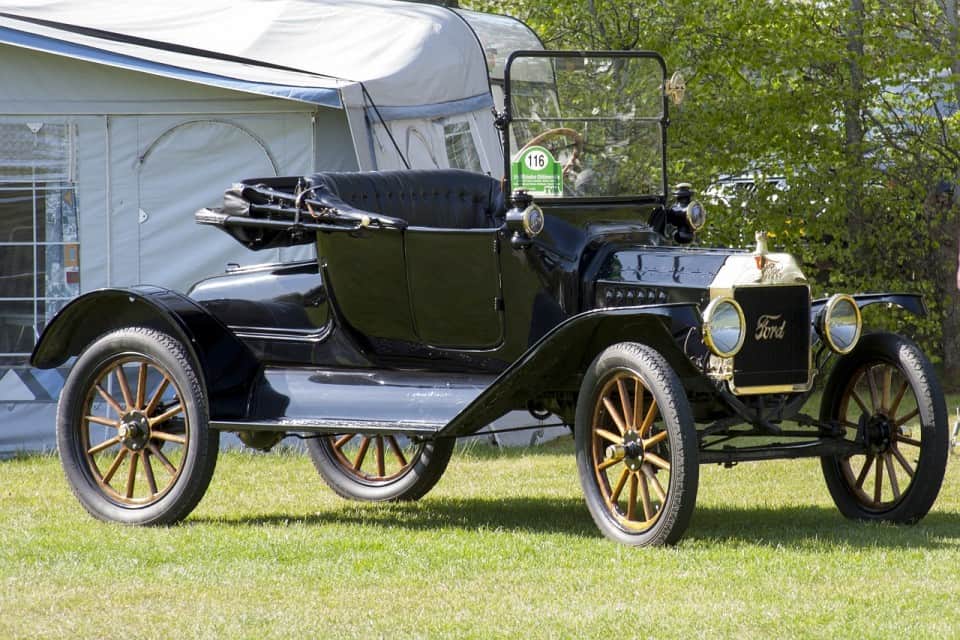
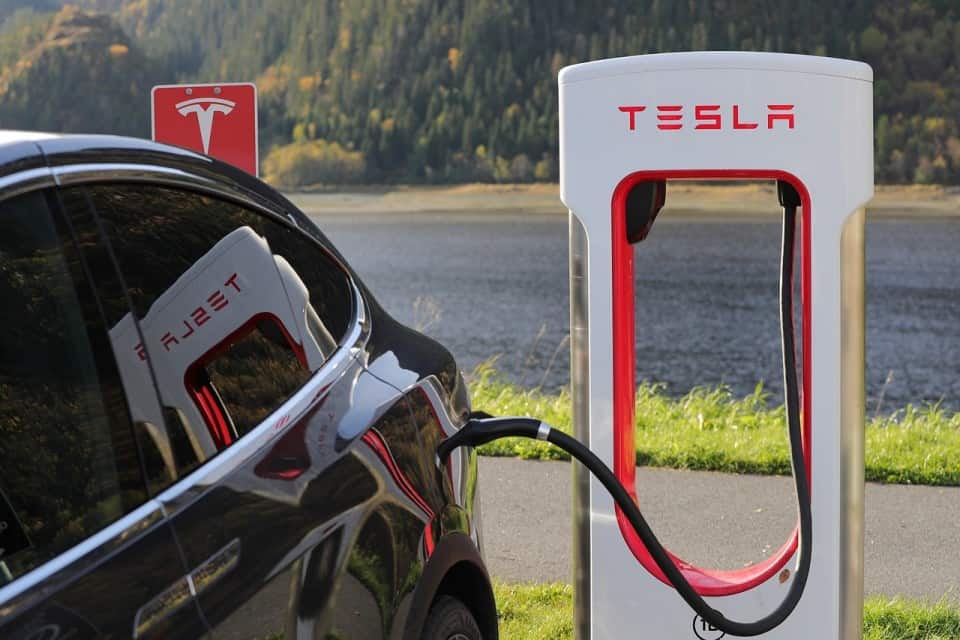
On a positive note, to move from Ford T to Tesla car has taken 111 years. Graham Bell invented in 1876 and first displayed at the Centennial Exposition, Philadelphia. The smartphone appeared in 2007. The first powered flight was in 1904 (Wright brothers) and the first commercial flight in Europe was in 1916. Digital Technologies are faster in being developed, and slower to being implemented mostly because of the organizational changes that they bring, regulations, data privacy, etc. Artificial Intelligence in Healthcare is not if it is when.
The healthcare industry is often at the forefront of innovation and technological advances due to the wealth of medical devices, equipment and processes that permeate the industry. But AI seems poised to transform the way we collect, understand and use data on patient health, healthcare services and historical health data to revolutionize medical diagnostics, treatment and research.
At the end of the day the main AI expected add value is to improve health outcomes, reduce health system costs, and improve patient experience. So how long must wait to see AI implemented in Healthcare..?
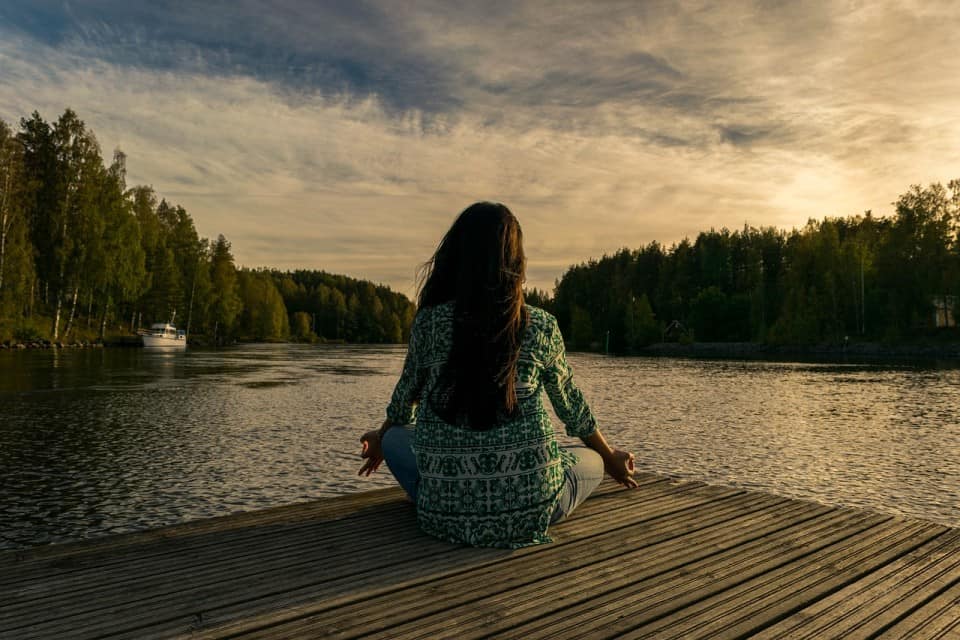
BIBLIOGRAPHY AND REFERENCES CONSULTED
- Alison Gopnik.Psychologist, UC, Berkeley; Author, The Gardener and the Carpenter
- The Role of medical doctor in the era of artificial intelligence. J Korean Med Assoc. 2019 Mar;62(3):136-139.
- Healthcare Informatics and Privacy: https://ieeexplore.ieee.org/abstract/document/8345561
- https://jkma.org/search.php?where=aview&id=10.5124/jkma.2019.62.3.136&code=0119JKMA&vmode=PUBREADER: Counterfactual Explanations without Opening the Black Box: Automated Decisions and the GDPR; https://arxiv.org/abs/1711.00399
- Proposed Regulatory Framework for Modifications to Artificial Intelligence/Machine Learning (AI/ML)-Based Software as a Medical Device (SaMD) – Discussion Paper; https://www.regulations.gov/document?D=FDA-2019-N-1185-0001
- Artificial intelligence and deep learning in ophthalmology. https://bjo.bmj.com/content/103/2/167; https://bjo.bmj.com/content/bjophthalmol/103/2/167.full.pdf
This is Part Two in a series of articles. Read Part One.